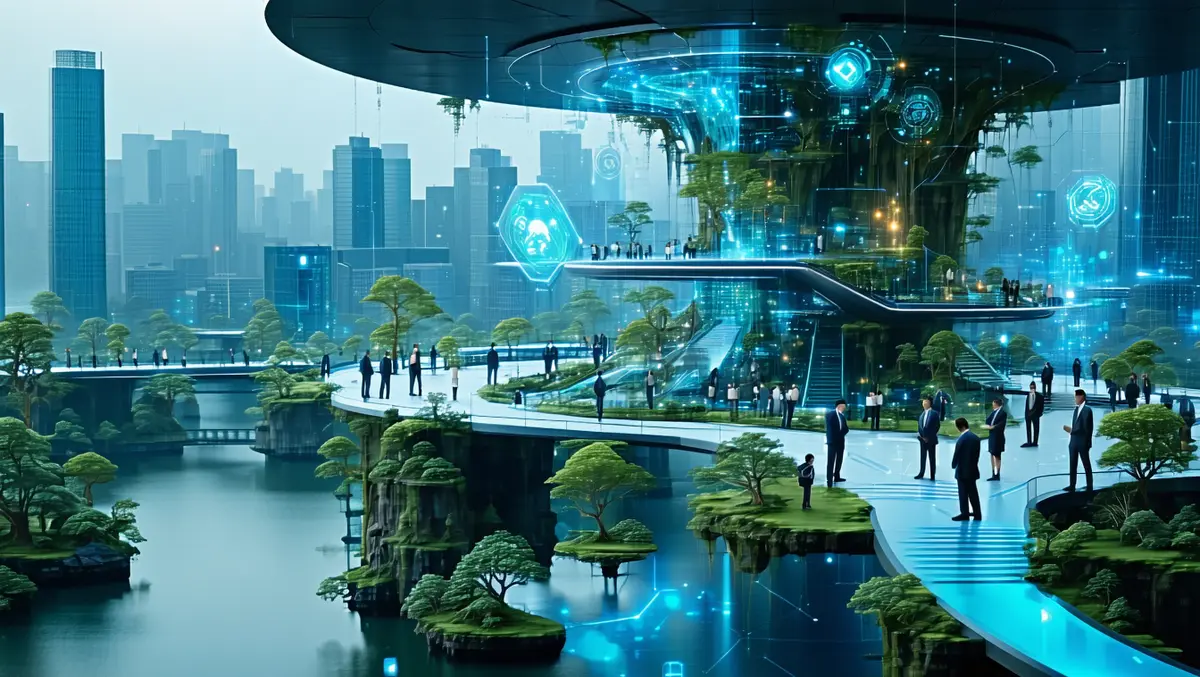
The cost of AI innovation is unpredictable. Here’s what APAC businesses need to know to stay competitive
In the months since the first release of ChatGPT, the tech industry has been in an AI frenzy. At the forefront, tech giants like OpenAI, Google, Meta, and Amazon are competing to build the generative AI models and infrastructure that will support a new wave of breakthroughs. Everyone else, from established enterprises to early-stage startups, are working to develop AI-backed solutions that will either transform existing offerings, or bring new features to market. Regardless of the size, all companies face the same harsh reality: AI tools are expensive, and the costs of building new AI-backed technologies are unpredictable.
As companies across the globe race to lead AI innovation and play in the AI game for the long haul, they need to start thinking strategically and making compromises. The businesses that win in our inevitable AI-enabled future won't necessarily be the ones with the best ideas; instead, the winners will be those that have figured out how to effectively balance cost and performance.
High costs, sudden shifts
Companies developing AI features are realising that leading-edge services like OpenAI ChatGPT-4 Turbo and Google Gemini 1.5 Pro come with hefty price tags. High-performing models such as Claude 3 Opus and GPT-4 Turbo are significantly more expensive than less advanced competitors. While newer releases like OpenAI GPT-4o offer better performance at lower costs, actual savings may be elusive as companies push these models into more complex use cases, ultimately consuming more tokens and incurring similar costs. As generative AI and LLMs evolve, the only constant may be high costs. For these technologies to play a lasting role in software stacks, they will need to deliver groundbreaking performance at more sustainable prices.
The true cost of AI
As companies develop new features using large language models (LLMs), they face three primary cost structures: Self-hosted/open source, Pay-as-you-go (PAYGO), and Provisioned Throughput Units (PTU). Self-hosting involves renting graphics processing units (GPUs) and running proprietary models, which, while avoiding popular vendors, incurs high costs and necessitates redundancies for reliability. Open-source models offer a more affordable alternative, but matching the performance of proprietary solutions like those from Google and OpenAI remains challenging, with significant cost savings generally achievable only at scale.
The PAYGO model, offered by numerous AI vendors, charges based on tokens processed, making it suitable for limited workloads and experimentation. However, performance can be inconsistent as traffic scales, leading some companies to switch between different endpoints to maximise efficiency. PTU, typically an upgrade from PAYGO, guarantees GPU access and performance within specific time frames but comes with steep costs. Companies often try to balance these by using PAYGO for experimentation and PTU for main loads. Ultimately, achieving top performance in AI is costly, requiring either the investment in self-hosted models or high expenditures for premium vendor endpoints.
How to control unpredictable AI costs
While industry giants can afford to pay top dollar for AI services, smaller and mid-sized enterprises must find strategic ways to achieve their desired outcomes without breaking the bank. To control unpredictable AI costs, companies should consider three key strategies:
- Narrowing the scope of AI features: Can significantly reduce spending. The most effective way to reduce spending is to choose use cases that offer the best value-to-LLM-query ratio, meaning they get the most value out of the fewest calls to an expensive LLM. This can be tricky and requires iterative optimisation of a prototypal feature and creative engineering, but there are definitely "low-hanging fruit" opportunities to reduce the number of expensive calls you need to make to the LLM to support a feature. For example, New Relic AI, a generative AI assistant, allows users to use a tool directly from the New Relic platform interface without a routing step, helping more engineers troubleshoot observability practices faster.
- Choose models for need, not novelty: Companies should choose AI models based on their specific needs rather than the allure of the latest innovations. While staying at the forefront of AI technology is crucial for some, many businesses can achieve their goals with slightly outdated models that still deliver impressive results at a fraction of the cost. As AI technologies and techniques continue to improve, older models can be optimised and enhanced to provide significant value, allowing companies to stretch their budgets further.
- Optimise for cost: Optimising for cost is essential when moving AI applications from prototype to production. While cutting-edge models are invaluable during the prototyping phase to validate the concept and ensure customer satisfaction, they may be too costly for long-term use. Instead, after confirming the value of a service with a powerful model, companies should focus on cost optimisation through improved prompting, retrieval-augmented generation, or supporting frameworks. This approach ensures that AI applications remain both effective and economically viable, allowing businesses to release and refine their offerings in a sustainable manner.
Embrace visibility
Despite the rapid evolution of generative AI technology, the fundamental questions underpinning the cost of AI are simple: How often do companies query an LLM and how much do those queries cost? By controlling these queries effectively and getting the most out of every call by adopting AI-supportive techniques such as retrieval augmented generation (RAG) and agent frameworks, companies can more reliably predict and lower their AI expenses
In the long term, generative AI features will truly become ubiquitous when we can reliably achieve the right balance between cost, performance, quality, and reliability. Observability helps companies maintain reliability, quality, and efficiency throughout all components of the AI technology stack, alongside services and infrastructure, so that they have the data they need to make decisions that limit expenses and maximise return on investment (ROI).
As the biggest players in tech continue to battle for AI market leadership, the technology landscape will remain unpredictable. Companies developing AI features need to be realistic about their goals and their needs. Those that can step back from the cutting edge will benefit consistently from more affordable AI services while still delivering value and innovation to their customers; they just need to identify the right use cases and use emerging AI-supportive techniques to get the most out of those LLMs. Companies that need to be at the forefront of AI or have good reason to use bleeding-edge models, on the other hand, will have no choice but to pay a steep price at the LLM layer. But for both types of organisations, observability needs to be a constant to enable proactive, data-backed decisions around AI investments.