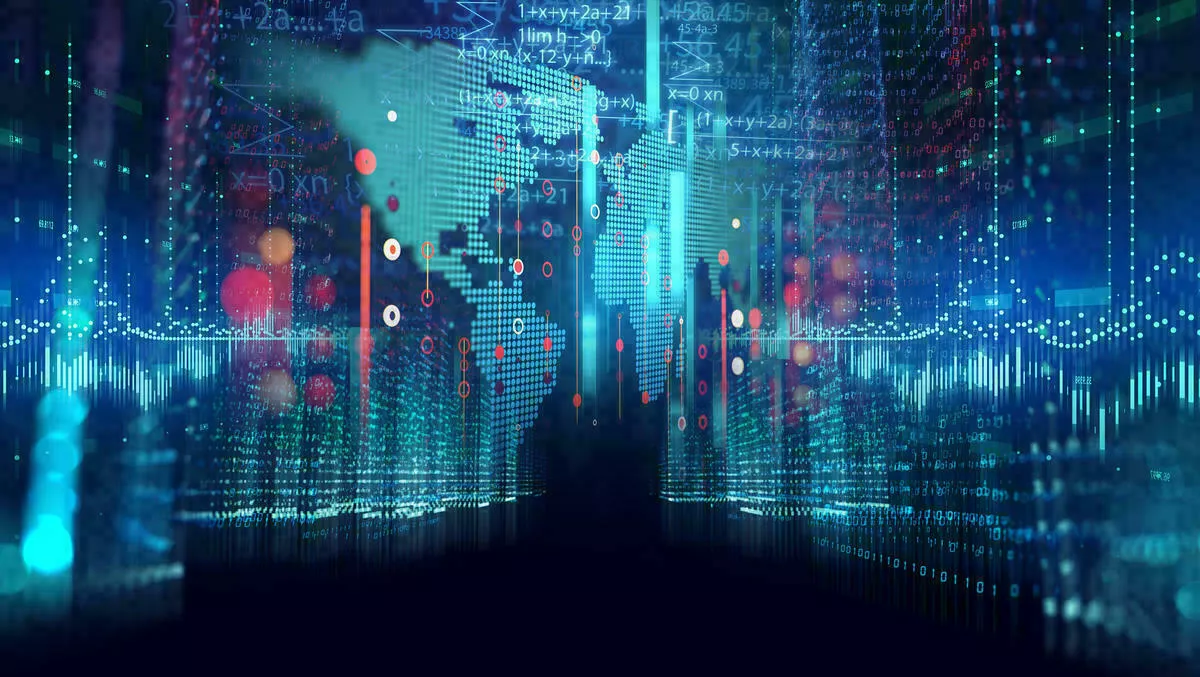
Asset management: moving to a smarter, data-driven future
Monitoring and managing ageing assets cost-effectively and in a timely manner are challenging organisations to identify more effective and efficient processes using intelligent, connected devices—and to do so with high safety, reliability and compliance.
Traditional and smart asset management have a common objective—aid in the reduction, minimisation and optimisation of asset lifecycle costs across all phases, from asset investment planning, all the way to operation and maintenance.
Optimising the costs associated with each phase will remain among the key objectives of an asset-intensive modern organisation.
At present, preventative maintenance schedules suggested by manufacturers are not enough to help companies avoid critical asset failures.
In order to improve customer satisfaction, organisations need to work towards avoiding unexpected downtime, managing asset risks and maintaining assets before failure strikes.
Reducing outages and shortening restoration times are among the most significant challenges faced across industries such as mining and resources, energy sourcing and distribution, and manufacturing - with facility and operations managers recognising the need for a mechanism to predict equipment failure.
The role of predictive analytics and virtualisation
More than ever before, facilities are leaning towards predictive analytics to extend the life of their assets, as well as to bring greater predictability to performance and organisational health.
By applying predictive analytics for smart asset management, organisations can realise asset lifecycle cost reduction while improving the accuracy of their decision-making, allowing them to plan and prioritise maintenance activities.
Industrial organisations these days employ a huge variety of data-driven, connected devices such as sensors, nodes, cameras, and access points which are constantly producing data.
As the organisation ingests this data, it can be either used for real-time reporting or stored for future use.
However, data that is stored away becomes less productive almost as soon as it reaches a data center – the more current the data, the more benefit can be derived from it.
But this is just part of the overall dataset required for this exercise.
In order to accurately predict equipment breakdown, companies need to combine this streaming data with operational data such as service life records, warranty data, and other information.
The streaming data coming in from sensors and connected devices and the operational data are of different types and reside in different source systems, further complicating the process of data integration and transformation.
Therefore, organisations who are looking to predictive maintenance for proactive field maintenance and optimising asset performance need to leverage data streams as soon as the data is available, in order to maximise its use.
Traditional data integration methods such as ETL often fall short, because bulk or batch data transfer is both slow and expensive, and also they replicate data into yet another repository to perform transformations.
Data virtualisation provides an excellent means of achieving this predictive maintenance, as it combines the streaming data with operational data to deliver meaningful information to business users in real-time, through interactive dashboards and reporting tools that sit on top of the data access layer.
Enterprise data does not get trapped in siloes, and individual departments can continue to maintain control over their portion of the company's data without affecting access to it for advanced analytics purposes.
By working proactively to virtually access and combine historic and current information to create predictive models for future events, organisations can:
· Improve customer satisfaction through uninterrupted operations: Customer satisfaction and the reliability of equipment are intrinsically linked.
· Prioritise maintenance activities and reduce TCO: Each asset has multiple associated costs, mainly in terms of procurement, installation, operations and maintenance, as well as failure and decommissioning. Unexpected failure cost is the leading expense component of any asset. These costs include the expense of the asset in service, collateral damage cost, regulatory penalty, disposal of a damaged asset, lost revenues and intangible costs.
By preventing key equipment failure, organisations can save a sizeable amount of money. Accurate modelling techniques utilise historical data from multiple sources, enabling the generation of predictions and risk scores. They also produce interpretable information to allow the understanding of implications of events, thus enabling the right response to be implemented,
· Optimise field crews with better route planning: A comprehensive understanding of asset health can serve organisations well in terms of work planning, prioritisation and scheduling. Unexpected equipment failure often requires reallocation of crews from other work locations to restore the outage, hiring of extra labour and often, an entire rescheduling of other planned maintenance activities.
· Improve overall safety and compliance: Predictive asset analytics pro-actively addresses potential safety risks by integrating data from multiple sources—SCADA (supervisory control and data acquisition), EAM-GIS (Enterprise Asset Management — Geographic Information System), online monitoring systems, weather channels along with nonoperational data, and so on. They enable organisations to identify safety risks and deploy suitable operational actions to mitigate these risks in a shorter span of time.
Alignment with business objectives is key
As organisations venture forward on their predictive analytics journey, the need to ensure that a predictive maintenance solution fits into the overall organisational strategy and future business requirements is vital.
Clearly define the immediate objectives of the solution; understand future business requirements and assess the scalability prerequisites to support additional applications.
Once these aspects are established, the data and analytics platform and statistical computational method for the solution will naturally flow.
Leveraging data virtualisation, companies can easily enable predictive analytics by combining the widest range of streaming and operational data and delivering it in real-time, making it the ideal logical data integration and delivery layer to feed visualisation and predictive analytical tools.
With these key technologies, manufacturing companies can boost their revenue with uninterrupted operations and improve their profit margins by saving on unintended downtime of key capital assets.